One Click for a Professional Look.
Still using selfies as your profile picture? An unprofessional image costs you opportunities. Change now and become a business pro instantly.
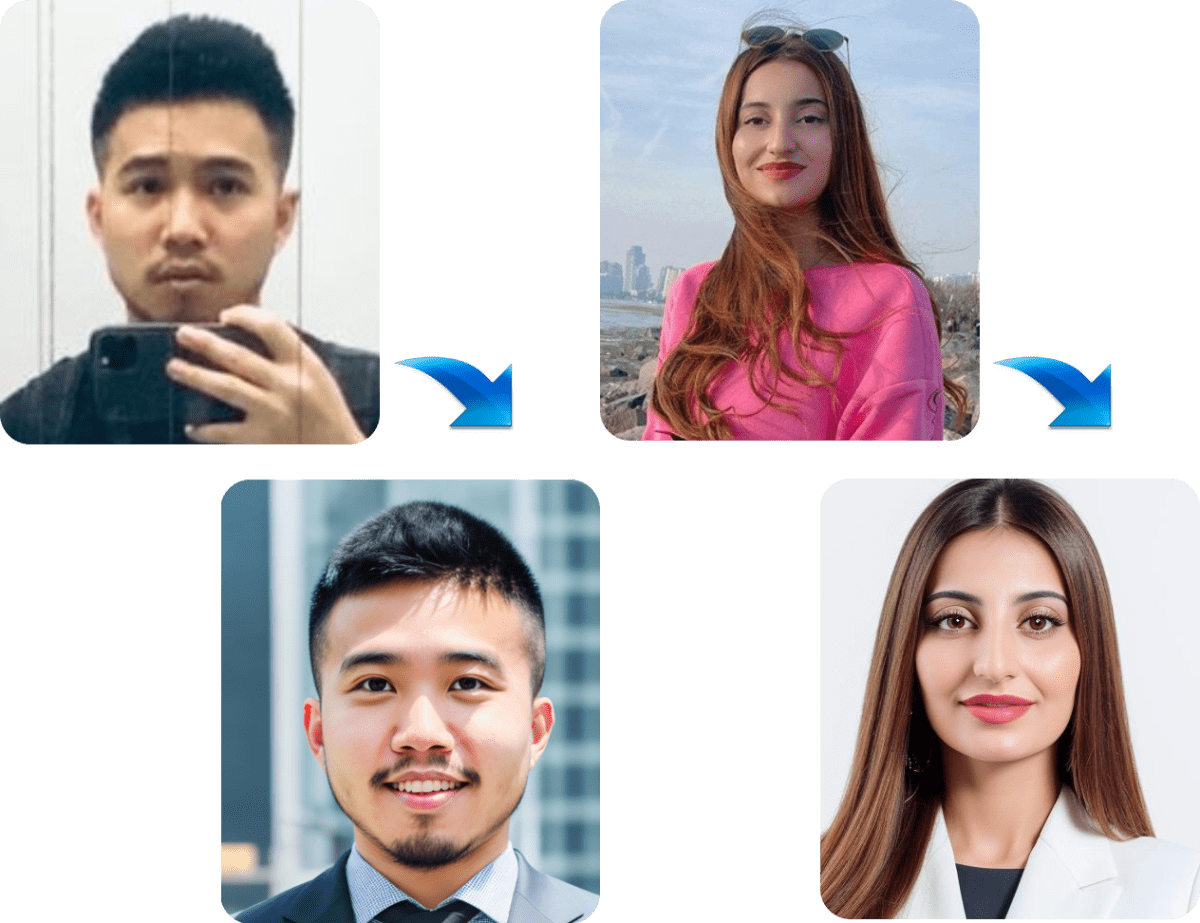
Why do few websites provide free AI services?
Especially for services like headshot generator that involve a large number of customized pictures, it is difficult to do it for free (unless there is a lot of capital to back it up). Of course, you can click on the top of this article to start using our service. In order to see the effect first and help you save unnecessary expenses, you can get a photo for free, but if you want to continue to generate more photos, you still need to pay the necessary fees to maintain the server's computing consumption. If you are interested, you can continue to the detailed principle introduction later.
Explanation of AI Computing Power
"AI computing power" refers to the computational capability used for performing artificial intelligence (AI) tasks and applications. This power allows users to create images, render, download, and participate in community activities within our tools.
Current Costs
At present, the costs we charge only cover the expense of generating images. The cost of training AI models is actually much higher than most people imagine. For example, a DGX A800 graphics card (since the A100 is banned by the US government and not available in some regions) costs around 1.8 million RMB per unit. Training unique models requires dozens of these cards, while fine-tuning a model requires at least one.
Post-Training Requirements
Even after training, generating images still requires significant graphics card resources. Currently, the price for a single 4090 graphics card from cloud providers is around 30,000 to 40,000 RMB. If purchased independently, it would cost nearly 200,000 RMB per unit. We use professional-grade graphics cards, which are even more expensive, and many units are needed to ensure smooth user experience.
Future Outlook
The AI industry needs the entire ecosystem to mature further, and various costs to decrease continuously. Only then can we offer more affordable and high-quality AI services.

Expand the field
The surge in generative AI is constrained by computing power. It uniquely improves directly with added computing resources. Typically, the investment in R&D is less directly tied to product value, but AI breaks this norm, making the cost of training and inference a key industry driver.
Supply and Demand in Computing Power
Though exact numbers are unknown, reliable sources indicate a severe supply constraint, with demand exceeding supply by tenfold. Thus, accessing computing resources at the lowest cost is crucial for AI companies' success. Many firms spend over 80% of their capital on computing.
AI Company Cost Breakdown
This article dissects AI company cost factors. Absolute numbers will change, but the access to computing resources remains a significant bottleneck. A useful framework for understanding this environment is provided.
Cost and Complexity of Generative AI Models
Generative AI models vary widely, with costs for inference and training depending on size and type. Popular models today are mainly Transformer-based, like GPT-3, GPT-J, or BERT. The computational cost is largely based on the number of model parameters and input/output tokens.
Tokens in AI Models
Tokens are short character sequences corresponding to words or parts of words. For GPT-3, the average token length is 4 characters. Forward passes (inference) in Transformers require about 2_n_p floating-point operations (FLOPs), where n is the token length and p is the number of parameters. Training involves additional operations, roughly 6*p FLOPs per token.
Memory Requirements for Transformers
Inference requires p parameters in memory, while training demands storing additional intermediate values. Using 32-bit floats, each parameter needs an extra 8 bytes. Training a 175-billion parameter model needs over 1 TB of memory, necessitating model partitioning across multiple GPUs.
Model Sizes and Computational Costs
The table below lists sizes and costs for common models. GPT-3, with 175 billion parameters, costs about 350 trillion FLOPs for a 1,024-token input/output. Training such models is among the most compute-intensive tasks undertaken by humans.
Computational Complexity and Cost
AI infrastructure is expensive due to the computationally intensive nature of the tasks. The complexity of generating text with GPT-3 far exceeds that of sorting database entries.
Hardware and Performance
GPUs, like NVIDIA A100, with 312 TFLOPS nominal performance, theoretically reduce GPT-3 inference to about 1 second. However, practical constraints like memory transfer and optimization techniques impact actual performance. For example, GPT-3's 175 billion weights (700GB) exceed any GPU's memory, requiring advanced techniques like partitioning and streaming.
Training Time and Cost
Training time for Transformers is about three times longer than inference. Training data sets are significantly larger than inference prompts, making training time exponentially longer. Training on single GPUs is impractical; instead, large data center clusters or cloud services are used, involving significant network considerations.
Cost of AI Infrastructure
GPT-3 inference on A100 costs between $0.0002 and $0.0014 per 1,000 tokens. Training costs are much higher, estimated at around $560,000 for a single run on A100 GPUs. These costs vary but indicate the significant investment required for AI model training.
AI Infrastructure Considerations
AI infrastructure investments are substantial, and this won't change soon. Training models like GPT-3 is one of the most compute-intensive tasks ever. Despite improvements in GPU speeds and training optimizations, AI's rapid expansion offsets these gains.
Infrastructure Choices: Internal vs. External
Many startups don't need to build their AI infrastructure from day one. Hosted model services, like OpenAI and Hugging Face, offer scalable solutions. These services are cost-effective and allow quick product-market fit without managing underlying infrastructure.
Cloud vs. Data Center
For most startups, the cloud is ideal due to lower upfront costs and scalability. Exceptions include large-scale operations or specific hardware needs not available from cloud providers. GPU price-performance comparisons and operational costs like power consumption are critical factors.
Comparing Cloud Service Providers
AWS, Microsoft Azure, and Google Cloud Platform offer GPU instances, but AI-specific providers like Coreweave and Lambda Labs also play significant roles. Pricing varies, with some providers offering lower costs through specialized data centers or arbitrage.
Hardware Comparison
High-end GPUs perform best but are costly. Choosing the right GPU type for your application can significantly lower costs. Considerations include memory requirements, hardware support, latency, and workload spikiness.
Model Optimization and Scheduling
Optimizing model runtime can lead to significant performance gains. Techniques include using shorter floating-point representations, quantization, and sparse matrix multiplications. Scheduling models to minimize weight swapping and utilizing high-performance interconnects are essential.
Future of AI Infrastructure Costs
Model parameters and GPU compute power have grown exponentially, but this trend may slow. Optimal parameter sizes are related to training data set sizes, and future AI growth will drive continued demand for faster GPUs. Efficiently managing these resources remains critical.
AI Infrastructure Choices and Considerations
Practical guidance for choosing AI infrastructure includes evaluating cloud vs. internal options and understanding GPU requirements. Hosted services offer cost-effective solutions for many startups, while large-scale operations may benefit from dedicated data centers.
More
Generative AI requires significant infrastructure investment. This situation is unlikely to change soon, as training models like GPT-3 remains one of the most compute-intensive tasks. Efficiently managing and optimizing AI resources is essential for future growth and sustainability.
Now you can go back to the top and see what AI tools we provide, which you can try for free.